Blogs / Addressing bias in AI-generated content
Addressing bias in AI-generated content
Admin / July 19, 2023
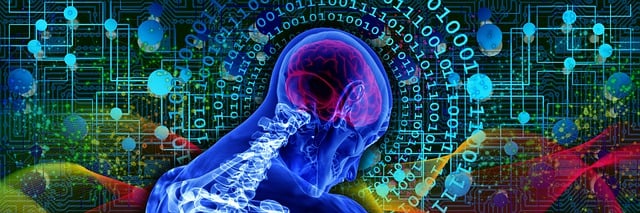
Artificial intelligence (AI) has become an integral part of our lives, with its applications ranging from virtual assistants to autonomous vehicles. However, as we increasingly rely on AI for decision-making processes, concerns about bias in AI-generated content have come to the forefront.
Bias in AI can have far-reaching consequences, affecting everything from hiring practices to online content recommendations. In this article, we will explore the importance of addressing bias in AI-generated content and highlight some of the efforts being made to mitigate this issue.
As technology continues to evolve, the need to ensure fair and unbiased AI-generated content is paramount. AI systems that are trained on biased data can inadvertently perpetuate and amplify existing societal biases, leading to unfair outcomes for marginalized groups.
For example, facial recognition technology has been shown to exhibit racial bias, resulting in the misidentification of individuals with darker skin tones. Similarly, AI algorithms used in hiring processes have been found to favor male candidates, disadvantaging women in the job market.
Addressing bias in AI-generated content is not just an ethical imperative; it is also crucial for building trust and ensuring the widespread adoption of AI technologies. If individuals don't perceive AI as fair, they may question its decision-making processes and be reluctant to embrace AI-powered solutions. Therefore, it is essential to proactively work towards minimizing bias and fostering transparency in AI systems.
Evaluating and Comparing Data
One of the ways to address bias is by improving the quality of data used to train AI models. The biased outcomes of AI systems often stem from biased training data. By ensuring that the data used to train these models is diverse, representative, and free from inherent biases, we can reduce the likelihood of biased output.
To achieve this, organizations are increasingly focusing on implementing ethical data collection practices, which involve collecting data that is reflective of the diverse perspectives and experiences of the users. Furthermore, data anonymization techniques can be employed to prevent the inclusion of personally identifiable information (PII), which can inadvertently lead to biased outcomes.
Ensuring Algorithmic Transparency in AI Decision Making
Another approach to addressing bias in AI-generated content is through algorithmic transparency. When you provide users with insights into how AI algorithms make decisions, organizations can help users understand the underlying processes and identify potential biases.
Algorithmic transparency can be achieved through techniques such as explainable AI, where AI models provide justifications or explanations for their decisions. This not only improves the interpretability of AI systems but also holds them accountable for their actions.
Incorporating Diverse AI Development Teams
Additionally, efforts are being made to diversify AI development teams. It is crucial to have diverse perspectives and experiences represented in the creation of AI systems to prevent the introduction of unconscious biases during the development process. Collaborative efforts involving individuals from diverse backgrounds can help identify and rectify biases that may be present in AI models, leading to fairer and more inclusive outcomes.
AI Government Policies and Regulations
The role of regulators and policymakers is also crucial in addressing bias in AI-generated content. It is imperative to have clear guidelines and regulations in place to prevent the deployment of biased AI systems.
Governments and regulatory bodies are increasingly recognizing the importance of this issue and are taking steps to ensure fairness and accountability in AI algorithms. Transparent reporting requirements, third-party audits, and the establishment of regulatory bodies dedicated to addressing AI bias are some of the measures being considered.
Constant Monitoring and Evaluation of AI Generated Content
Moreover, ongoing monitoring and evaluation of AI-generated content are necessary to identify and rectify biases that may emerge over time. Bias can evolve as societal norms change, and it is crucial to continuously assess AI systems for any bias that may have crept in.
This requires organizations to employ dedicated teams that regularly analyze the output of AI systems, conduct bias audits, and actively work towards mitigating biases whenever they are identified.
Final Thought
In conclusion, addressing bias in AI-generated content is crucial for ensuring fair outcomes, building trust, and fostering widespread adoption of AI technologies. By improving the quality of training data, promoting algorithmic transparency, diversifying development teams, involving regulators, and conducting ongoing monitoring and evaluation, we can take significant strides toward mitigating bias in AI.
It is an exciting time as we harness the transformative power of AI while also being cognizant of its potential pitfalls. By proactively working towards a fair and inclusive AI ecosystem, we can create a future where AI-generated content is a powerful tool for positive change.